Gen AI Optimizer
The Enterprise LLM Optimization & Evaluation PlatformThe most widely utilized and trusted LLM optimization platform for fine-tuning LLMs for mission critical use cases leveraging subject matter experts to optimize and tag data to reduce hallucinations.
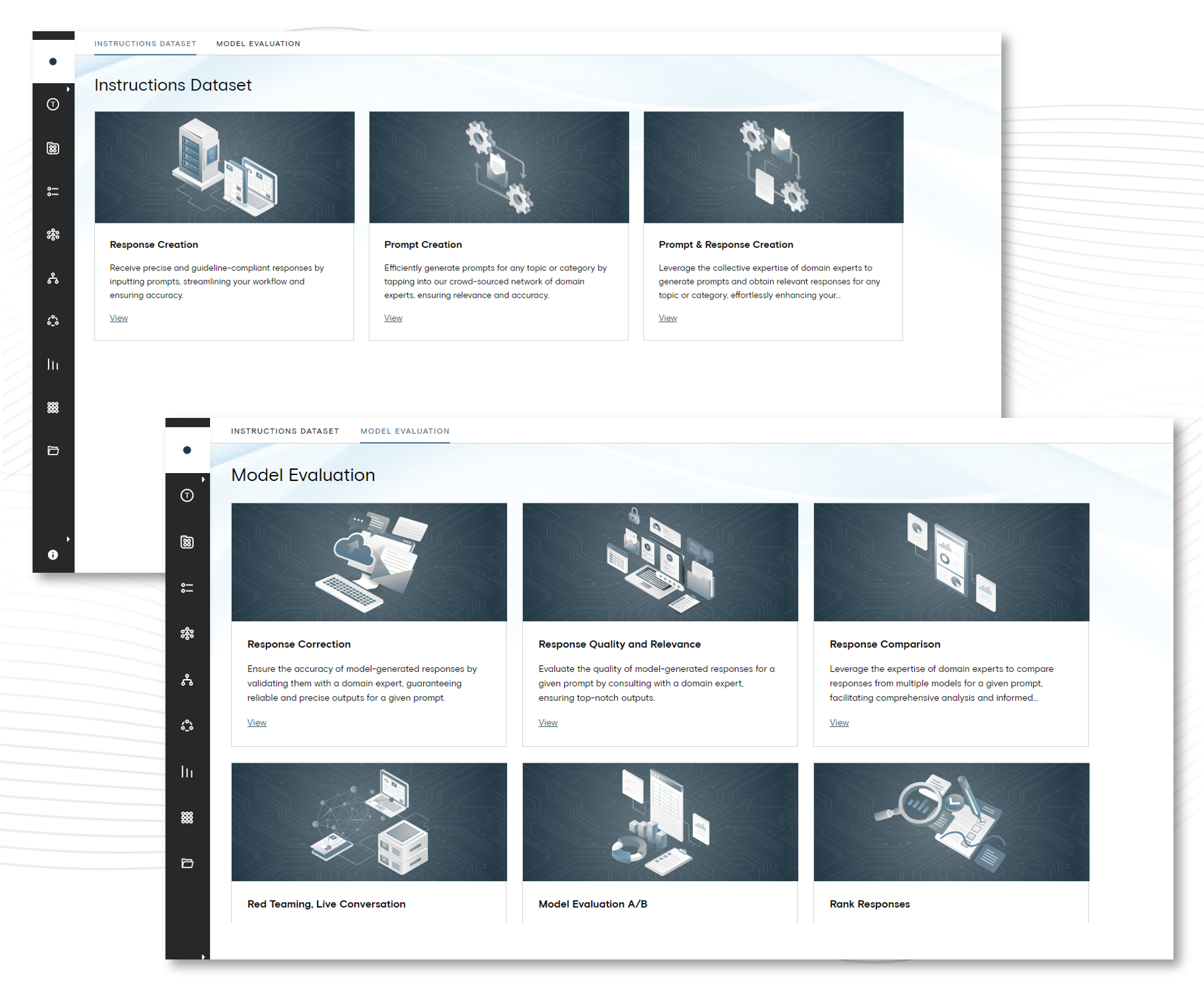
Unmatched LLM Fine-tuning Capabilities Powering Gen AI Initiatives Around the Globe
LLM Interaction Designer
Drag-and-Drop designer for crafting rapid, optimized ways to interact with 1 or multiple LLMs for comparison, evaluation, and fine-tuning for industry specific interfaces for any use case and any data type. Reduces technical barriers to designing optimized data transformation interfaces for enhanced efficiency and increased quality across data labeling and model evaluation jobs.
Optimize LLMs with Fine-Tuning
Create prompt and response text pairs to fine-tune the model. Set specific parameters for the text, such as length range and rich formatting, to ensure that the prompt and response pairs meet your requirements and effectively trains the model.
Compare LLMs A/B Testing
Empower your end operators to craft drag-and-drop interfaces to interact and capture feedback to both test and evaluate an LLM for operational use while capturing that feedback in a structure that can be used to optimize the LLM for mission specific needs.
Multi-Modal Model Optimization
Teach multi-modal models to understand what’s going on in an image and respond to different instructions and questions about it with customized prompts based on custom prompts based on image input
Better Metadata = Better RAG
Enrich your raw documents before creating chunks. Add labels such as categories or intents to raw documents to increase the accuracy of an RAG application against mission specific data.
Refined Chunks to Optimize RAG
Review chunks and validate their semantic completeness, relevance, and absence of truncation through comparison with the source raw document. Leverage optimized chunk text to create better RAG applications on complex mission data.
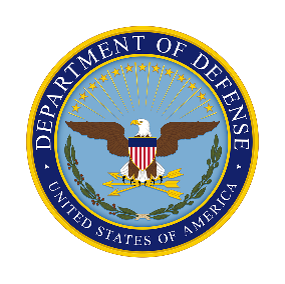
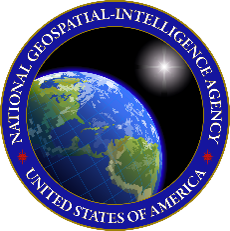
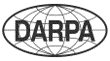

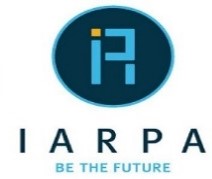
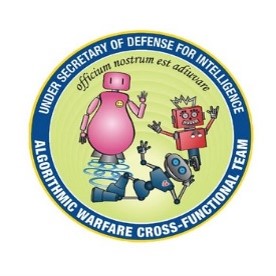
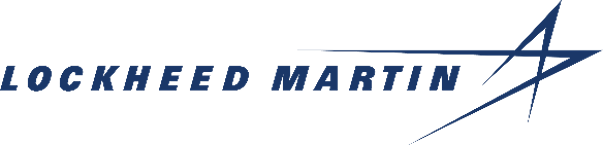
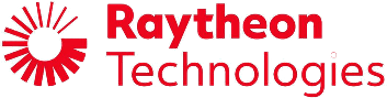
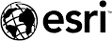
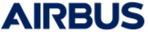
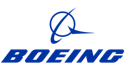
